6 Key Phases of Successful First-Party Data Activation
Nick Bennett
March 23, 2023
When it comes to data activation, turning raw data into actionable insights doesn’t just happen with a wave of a magic wand. And while some may have you believe it can happen with an “if you build a complex infrastructure, it will come” scenario, or worse a “you NEED a complex infrastructure, for it to come” mindset, it’s not that simple, and at the same time, it’s not that hard.
Successful first-party data activation demands planning, identifying metrics, strategizing, resource allocation, testing and learning, and constant evaluation.
Before we jump in, you may be wondering why we’re specifying first-party data (FPD) activation. The answer is simple. With the combination of recent and upcoming technical & regulatory industry changes (e.g., CCPA, GDPR, IDFA removal, cookie deprecation), everything data– from collection to storage to digital measurement approaches needs to be firmly rooted in FPD in order to be future-proof against the changing digital ecosystem.
First-party data activation is an initiative, and as with most initiatives, to be successful you need a plan that involves setting goals and objectives and answering some basic questions like:
- Who needs to be involved?
- What data do I have?
- What metrics am I trying to move the bar on?
- How will I measure effectiveness?
- What does a good foundation require?
To fully answer these and other questions, and to identify requirements for a successful data activation initiative, we’ve identified 6 key phases:
6 Key Phases of Data Activation
- Establishing a good foundation
- Establishing criteria for success
- Identifying the necessary data
- Bringing the data together at the right point for activation
- Activation testing and measuring success
- Growth and resilience
The best way to describe these phases is with a specific example.
Let’s say you are an online retailer. Your business objective is to increase high lifetime value (LTV) customers and your marketing goal is to increase the percentage of high LTV customers.
To begin you need to:
- identify who your customers with high LTV are, and
- be able to understand their behaviours so you can
- determine which customers “look like” high LTV customers, but haven’t yet achieved high LTV.
One of the tactics you might take is to run a campaign against an audience segment that looks like your high LTV customers but hasn’t achieved high LTV yet. To do this, you’ll need two datasets: digital analytics and customer data. Then, you can do some segmentation and behavioural analysis to identify an audience of people who share the same behaviours as high LTV customers but haven’t yet achieved high LTV. Once you have those audiences, you’ll want to activate against them, probably in your advertising tools.
There are a couple of ways you can do this.
Something I think that is very important to note is that you don’t necessarily have to have a big complex structure like a Google Cloud infrastructure, a CDP (customer data platform), or a tool like Liveramp to activate against certain kinds of data. It can be as simple as bringing a few key elements into your analytics tool and doing some segmentation and activation directly in the analytics store. This approach has some quick and easy wins but it does have some limitations. For example, due to regulations, you can’t load PII into Google Analytics which means there are certain kinds of analysis you won’t be able to do because of the limitations of the tool itself.
Another way is to bring your behavioral data and your customer data together in Google Cloud. Doing this will allow you to apply more advanced techniques to do segmentation of behavioural analysis and you can then share the outcomes of this analysis back to the GMP stack, or your email tool, etc to activate against the campaign. This option allows you to bring more data together because it doesn’t have restrictions on PII and offers more sophisticated algorithms.
If you don’t already have a complex infrastructure in place you can start activating your data with option one until you come to the point where you want to upgrade your infrastructure or iterate on the infrastructure you have in order to accommodate the growing breadth and depth of analysis you want to do.
You may find investing in a more complex infrastructure makes sense for what you want to achieve, but I want to stress that it doesn’t necessarily have to be the first iteration of things you do to be able to start activating your data.
Figuring out how complex your needs are will come out of your work to establish a good foundation (phase 1) because it requires you to do an assessment of the infrastructure you already have by asking and answering questions like
- Where does the data live?
- Who owns it?
- What is your primary source of truth?
Primary Source of Truth
There are a lot of places within a business where customer data might show up, for example, an invoicing system, a marketing email list, an order management system, etc. But, customer data lives in the CRM, making it the source of truth. All of the other places may have access to some pieces of the data but it lives in the CRM.
When you start talking about trying to combine your data sources, it’s important to ensure you’re merging data from the primary source of truth so you’re not inheriting secondhand data or data that’s been sub-processed. So, if you’re employing finance data, you’re pulling it from the finance system, if you’re employing CRM data, you’re pulling it from the CRM system. etc.
Bringing the Data Together
When it comes to bringing data together effectively you need to know things like, where does it live? What does it look like? What types of common data points do you have between these systems?
Back to our example:
If you’re a retailer who wants to join CRM data with web behavioral data, the ideal situation occurs if you have a site where a customer has to log in to make purchases.
If a customer has to log in, then you know who they are because you have a user ID in your internal systems. If you have user IDs, then you can implement the user ID feature in Google Analytics. Then, all of the customer’s behaviours as they interact with your website or app are associated with the user ID in a way the business understands. Now, you can easily connect the web data of that customer to their CRM record because of this common user-id “key”.
A lot of the heavy lifting around data integration, data joining and deduplication comes down to finding these common points so you can connect the right behavioural data to the right CRM contact. A lot of people get hung up on trying to find these universal keys that let all of this data come together in a consistent way.
When working with clients on foundational deployments we ensure the Google Analytics data contains these “keys”.
So when you’re identifying product IDs, you’re making sure what you’re using matches what is being used in the inventory system and the merchandising system. The product ID should be the same throughout all three systems so when you come to the point of joining these data sets together you’ll have a much easier time.
Bringing the Data Owners Together
It’s important to remember that all of these different datasets have different organizational owners. So one of the core elements of a successful first-party data activation initiative is bringing those data owners together and helping them understand why there’s value in joining these data sets. Once all of the owners appreciate this value you can all move towards data architectures that make bringing the data sets together easier.
Trusting Your Data
When building your foundation, an important question to ask yourself is, “Do you trust the data you are using?” Or, “What is the quality of my first-party data”. The answer is important, because as they say, garbage in, garbage out. If your analytics data isn’t very good, then doing behavioral analysis on that data isn’t going to yield high-quality results. If your CRM is a mess, then joining CRM data with behavioural data is just going to make a bigger mess. Assessing how strong the pillars of data on which you are building your first-party data initiative is key.
Growth & Resilience
In order for data analytics to be successful in driving growth for a business its foundation must also incorporate resilience. A resilient system is one that is robust to unexpected change. It’s an overarching principle that says when you architect the infrastructure you are going to use to measure things, you’re thinking about:
- What happens if you need to change something you couldn’t predict you would need to change?
- What happens if the behavior of something changes in a way you can’t predict?
- How easily can you recover from that disruptive change and continue fulfilling the business objectives you’re trying to fulfill?
The infrastructure you build needs to be capable of providing the insights to drive activations you want to be able to do AND the activations that you might want to do next. It’s an inherently iterative process, not a one-and-done solution.
There is a lot of value in growing your infrastructure to match the growth of your organization’s sophistication.
For some people, the requirements are going to be fairly complicated. For example, if the use case is “I want to do real-time personalization and orchestration of very specific campaign messages to individual behaviors based on what people are doing from moment to moment as they interact with my brand,” you’ll need a fairly sophisticated infrastructure to be able to do that.
If you’re trying to get a handle on “I’d like to be able to better address certain segments of my customer population more diligently with more focused campaigns,” you don’t necessarily need a complex infrastructure. You just need to be able to bring together the data that lets you understand behavior with the data that lets you understand your customer segments, and if you can do that, then you can run a long way with relatively simple integrations.
The Napkin point of view is that one size does not fit all, and it is possible to do these things in steps. There is value to be had even in some relatively simple data integrations.
Going through these iterations and having an informed decision about what you’re going to do next, why you’re going to do it, and what value you’re going to get out of it, is the way forward to continue to capitalize on the data you have in ways that are measurably improving your business performance.
More Resources
If a first-party data initiative is something your organization would like to learn more about, here are some resources we recommend:
Debunking First-Party Data Activation Myths (article)
5 Key Characteristics of a Solid Analytics Foundation (article)
How to Build a Future-proof Media Measurement Plan (article)
How to Identify and Leverage First-Party Data (on-demand webinar recording)
3 First Party Data Activation Trends you Need to Know About (article)
And of course, you can always reach out to us.
Latest Articles
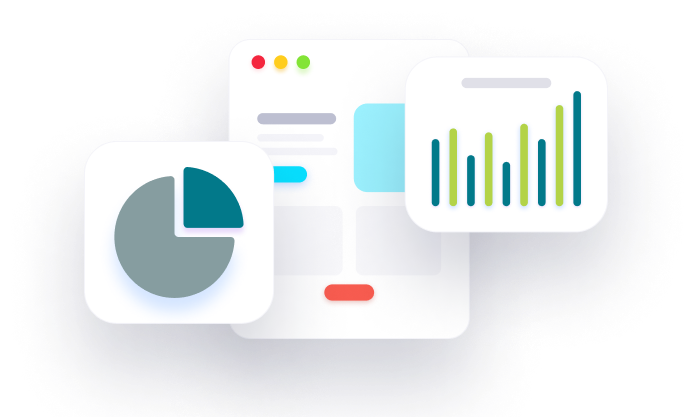