How Marketing Mix Models Can Optimize Marketing Budgets
Unlocking Marketing Efficiency: A Comprehensive Exploration of How Marketing Mix Models Optimize Budgets and Essential Starting Points
Abstract:
This post delves into marketing analytics, focusing on the pivotal role of Marketing Mix Models (MMM) in optimizing marketing budgets. By investigating the fundamental principles and methodologies behind MMM, this study aims to provide actionable insights into how businesses can enhance their marketing efficiency. Essential prerequisites and considerations for implementing MMM will be explored, offering a comprehensive guide for organizations aspiring to harness the full potential of this analytical technique.
Introduction:
Marketing Mix Modelling (MMM) is an advanced analytics technique used by businesses to evaluate the impact of different marketing activities on overall sales or key performance indicators. These models assist in optimizing marketing budgets by providing insights into the effectiveness of various marketing elements and their contribution to the overall success of an organization. The primary objective of Marketing Mix Modeling (MMM) is to improve the efficiency of marketing budgets and strategies to increase sales. Typically, marketing budgets account for 5% to 15% of a company's total budget, but for newer businesses, this figure may reach 20%. The impact and effectiveness of these budgets can be significantly enhanced by optimizing expenditures using MMM. Here's how Marketing Mix Models can optimize marketing budgets and the requirements to get started.
Benefits of Marketing Mix Models for Budget Optimization:
Businesses today commonly use multi-channel initiatives to expand their operations. These initiatives involve various marketing channels including PPC, PR, content creation, organic and paid social media, co-selling, and promotions. While using multiple channels can increase audience reach and business growth, it also means marketers have more data to monitor and analyze. This is where Marketing Mix Modeling (MMM) comes in. MMM allows for the measurement of each marketing channel's impact on conversions, enabling a precise evaluation of the return on investment (ROI) for different campaigns and channels.
Allocation of Resources:
-
- Identify the most effective marketing channels and tactics.
- Allocate the budget based on the channels that contribute the most to sales or desired outcomes.
Performance Measurement:
-
- Evaluate the performance of each marketing element.
- Understand the return on investment (ROI) for different channels.
Optimization of Campaigns:
-
- Adjust campaigns in real-time for better performance.
- Identify underperforming elements and optimize or eliminate them.
Forecasting:
-
- Use historical data to predict future performance.
- Plan budgets more accurately by understanding the expected impact of various marketing activities.
Efficiency Improvement:
-
- Avoid over-investing in less effective channels.
- Improve the efficiency of marketing spending by focusing on high-impact strategies.
What You Need to Start:
Data:
All Marketing Mix Models employ three general categories of data, as outlined below, and possess the following characteristics:
-
-
- Time-series format
- As granular as possible (daily data is typically sufficient)
- Structured, machine-readable format
-
The primary objective of Marketing Mix Modeling is to comprehend the impact of marketing activities on sales. Therefore, having granular and high-quality sales data is fundamental for constructing all models. When deciding on the data to be utilized, the following considerations are crucial:
Dimensions and features of the sales data. Typically dimensions/features include:
-
- Geography (for example, 'country')
- Sales channel ('brick & mortar', 'online', ..)
- Product Group
- For FMCG/brands with multiple brands: Brand
Unit of the sales data:
-
- Volume
- Value
Source of the sales data:
-
- Conversion data from online platforms (e.g., Google Analytics)
- Sales data from an ERP or similar company-owned system:
- Sales data collected by a 3rd party
Marketing Mix Models analyze marketing activities to measure the impact of different marketing efforts on sales. The accuracy of data pertaining to a particular media channel directly affects the model's ability to estimate the influence of that channel. Here are the key dimensions that should be considered:
1. Media hierarchy-
-
- Media Type: Online media, Offline media, Own media
- Media Groups within Media Types: TV, OOH, Radio, Display, Search, etc.
- Individual media channels with Media Groups
-
-
-
- Campaign types: brand, tactical, seasonal, etc.
- Campaign names of individual campaigns (different for each company)
-
The dataset should include all media:
-
-
- Investments
- Exposure metrics (such as TRPs for TV, Impressions for Display)
- Other metrics relevant to the medium (e.g., clicks)
-
3. Non-marketing variables
Certain variables can have a significant impact on company sales, even if they are not directly related to marketing efforts. It is essential to consider the influence of these variables to avoid mistakenly attributing their positive or negative effects to media in the Marketing Mix Model. Some examples of these variables include:
-
- Pricing & discounts, Distribution network, Macroeconomics, Weather, Competitor activities, COVID-19, Holidays, and Seasonality
- Pricing & discounts, Distribution network, Macroeconomics, Weather, Competitor activities, COVID-19, Holidays, and Seasonality
Note: To make a decent market mix model, you need at least 3 years of data, preferably weekly data. One can also interpolate daily data to weekly data.
Types of MMM Models
Marketing Mix Models (MMM) encompass a range of methodologies to analyze and attribute the impact of marketing endeavours on business outcomes. Let's delve into some prominent MMM variants and evaluate their advantages and disadvantages:
1. Linear Attribution Models:
Pros:
-
-
-
-
- Simplicity: Easy to understand and implement.
- Uniform distribution of credit across all touchpoints.
-
-
-
Cons:
-
-
-
-
- Oversimplification: Doesn't account for variations in impact among different channels.
- May not reflect the actual customer journey.
-
-
-
2. Time Decay Attribution Models:
Pros:
-
-
-
-
- Recognizes that the impact of touchpoints may diminish over time.
- Gives more credit to recent interactions.
-
-
-
Cons:
-
-
-
-
- Still needs to be more concise about the complex nature of customer journeys.
- Assumes a linear decay pattern which may not be accurate in all cases.
-
-
-
3. U-Shaped (Position-Based) Attribution Models:
Pros:
-
-
-
-
- Recognizes the importance of both early and late touchpoints in the customer journey.
- Provides a balanced view of the entire customer journey.
-
-
-
Cons:
-
-
-
-
- Still assumes a fixed distribution of credit and may not capture variations accurately.
- The weight assigned to touchpoints is arbitrary.
-
-
-
4. Algorithmic Attribution Models:
Pros:
-
-
-
-
- Uses machine learning algorithms to dynamically assign weights based on actual data patterns.
- More flexible and adaptive than rule-based models.
-
-
-
Cons:
-
-
-
-
- Complexity: Requires expertise in machine learning and data science.
- Interpretability: The inner workings of the algorithm may be challenging to explain.
-
-
-
5. Data-Driven Attribution Models:
Pros:
-
-
-
-
- Utilizes historical data to understand the actual impact of each touchpoint.
- Adapts to changes in consumer behaviour over time.
-
-
-
Cons:
-
-
-
-
- Highly dependent on the quality and accuracy of historical data.
- May require significant computing resources for analysis.
-
-
-
6. Marketing Mix Modeling (MMM):
Pros:
-
-
-
-
- Takes a holistic approach, considering various marketing elements and their interdependencies.
- Incorporates offline and online channels for a comprehensive view.
-
-
-
Cons:
-
-
-
-
- Complexity: Requires advanced statistical analysis and expertise.
- Time Lag: Results may not be immediately available due to the need for historical data.
-
-
-
7. Shapley Value Attribution Models:
Pros:
-
-
-
-
- Based on cooperative game theory, providing a fair value distribution among touchpoints.
- Considers all possible combinations of touchpoints in the customer journey.
-
-
-
Cons:
-
-
-
-
- Computationally intensive, especially with a large number of touchpoints.
- Requires a clear definition of the value being attributed.
-
-
-
8. Markov Chain Models:
Pros:
-
-
-
-
- Models customer journeys as a sequence of states and transitions.
- Considers the probabilistic nature of transitions between touchpoints.
-
-
-
Cons:
-
-
-
-
- Assumes a Markovian property, meaning that future states depend only on the current state (memoryless), which may not hold in all cases.
- Requires a significant amount of historical data for accurate modelling.
-
-
-
The effectiveness of these models can vary depending on the business, customer journey complexity, and data availability. Often, a combination of models or a customized approach may be necessary to precisely capture the dynamics of a particular marketing ecosystem.
Steps to apply marketing mix model
- Identify the relevant factors that need to be analyzed, like demographics, seasonality, pricing strategies, promotional campaigns, and customer loyalty programs.
- Gather data from past marketing activities or industry research.
- Build the model based on the gathered data and create a mathematical model that reflects your product or service’s marketplace.
- Test various scenarios and refine your model by changing the mathematical equations based on the scenario analysis.
- Present results to stakeholders so they can make informed decisions about their marketing investments.
How do we interpret the results from MMM?
When it comes to interpreting the results of a Marketing Mix Model (MMM), the key lies in comprehending the effect that different marketing components have on a particular business outcome, typically sales. Here is a guide to help you interpret the results:
Coefficient Values:
-
- Positive Coefficients: A positive coefficient for a marketing variable indicates a positive impact on the outcome variable (e.g., sales). An increase in spending on that marketing channel is associated with an increase in sales.
- Negative Coefficients: Conversely, a negative coefficient suggests a negative impact. An increase in spending on that channel is associated with a decrease in sales.
The magnitude of Coefficients:
-
- Larger Magnitude: The magnitude of the coefficients indicates the strength of the impact. Larger coefficients suggest a more significant influence on the outcome variable.
Statistical Significance:
-
- P-Values: Check the statistical significance of coefficients using p-values. A low p-value (typically < 0.05) indicates that the relationship between the marketing variable and the outcome variable is statistically significant.
Overall Model Fit:
-
- R-squared Value: Assess the overall fit of the model with the R-squared value. A higher R-squared value indicates a better fit, implying that the model explains a larger portion of the variability in the outcome variable.
Attribution and Contribution:
-
- Contribution to Sales: Examine how each marketing channel contributes to overall sales. Some channels may have a more substantial impact than others.
Budget Allocation:
-
- Optimal Budget Allocation: If ROI information is available, allocate budget to channels that provide the highest return on investment. The model can guide optimal budget allocation for maximizing outcomes.
Sensitivity Analysis:
-
- Conduct Sensitivity Analysis: Assess how changes in marketing spend for each channel impact the overall outcome. This helps understand the elasticity of each marketing element.
Time Lag Effects:
-
- Consider Time Lag Effects: Understand if there are time delays in the impact of marketing activities on outcomes. Some channels may have a delayed effect on sales.
External Factors:
-
- Consider External Factors: Be aware of external factors such as seasonality, economic conditions, or competitive actions that may influence the results.
Continuous Monitoring:
-
- Regularly Monitor and Update: Marketing environments change, and consumer behaviors evolve. Continuously monitor the model's performance and update it to reflect the current landscape.
How does MMM look in a cookieless world?
In an era where privacy concerns and regulatory changes have disrupted traditional tracking methods like third-party cookies, marketers must adjust their approach to Marketing Mix Models (MMM) for digital marketing and advertising. Here's a glimpse into how MMM can be applied, specifically when leveraging Google Analytics data and marketing through Google Ads.
- Focus on First-Party Data:
The rise of limitations on third-party cookies has placed a greater emphasis on the utilization of first-party data. Google Analytics remains capable of capturing first-party data, specifically user interactions on websites, which in turn offers valuable insights into user behavior.
- Google Analytics Integration:
Google Analytics is essential for obtaining valuable insights into website interactions, user journeys, and conversions. By incorporating Google Analytics data into the MMM model, a holistic understanding of online user behavior can be achieved.
- Attribution Modeling in Google Analytics:
Google Analytics offers multiple attribution models, including last click, first click, and linear attribution. These models help analyze the impact of various touchpoints on conversions and can be seamlessly integrated into broader MMM analysis.
- Custom Channel Groupings:
Marketers can employ custom channel groupings in Google Analytics to classify traffic sources according to their distinct business models. This enables MMM to evaluate the impact of various marketing channels on overall business performance.
- Event Tracking for Key Actions:
Implementing event tracking in Google Analytics enables marketers to capture user interactions, including form submissions, video views, and other significant actions. These events can be analyzed within the MMM model to gain insights into their influence on business objectives.
- Google Ads Conversion Tracking:
Google Ads provides conversion tracking tools that enable advertisers to measure the success of their campaigns. Integrating Google Ads conversion data into MMM helps assess the contribution of paid search campaigns to overall business performance.
Google Ads offers conversion tracking tools to help advertisers measure the effectiveness of their campaigns. By incorporating Google Ads conversion data into MMM (Marketing Mix Modeling), the impact of paid search campaigns on the overall business performance can be evaluated.
- Incorporating Offline Data:
MMM should not be limited to online data. Integrating offline data sources, such as CRM or sales data, into the model to provide a more complete picture of the customer journey and business impact is important.
- Machine Learning for Predictive Analysis:
In the absence of individual-level tracking, machine learning algorithms can help predict and attribute the impact of marketing activities. These algorithms can analyze patterns in aggregated data to understand how different factors contribute to outcomes.
- Privacy-Compliant Analysis:
Ensure that the use of Google Analytics data and Google Ads information adheres to privacy regulations. This involves anonymizing and aggregating data to protect user privacy.
Challenges:
Limited User-Level Tracking: With restrictions on cookies, user-level tracking is limited. This challenges the granularity of data available for analysis.
Data Integration Complexity: Integrating data from various sources, such as Google Analytics, Google Ads, and offline sources, can be complex. Proper data governance and integration processes are crucial.
Adapting to Changes: Given the dynamic nature of privacy regulations, marketers need to stay informed and be agile in adapting their MMM strategies to comply with evolving standards.
Adapting Marketing Mix Models for digital marketing and advertising in a cookieless world involves leveraging available first-party data, integrating various sources, using privacy-compliant analytics, and incorporating machine learning for predictive insights. This ensures marketers can still derive valuable insights from their digital marketing efforts despite the changing landscape.
Latest Articles
Ready to grow your company to the next level?
Contact Napkyn to discuss the tailored solutions we can implement for your team.
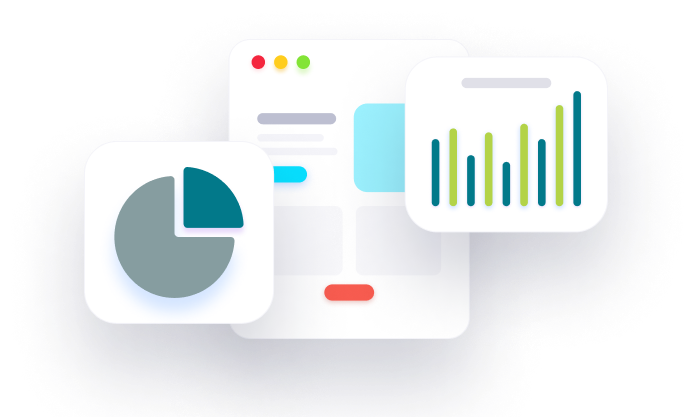